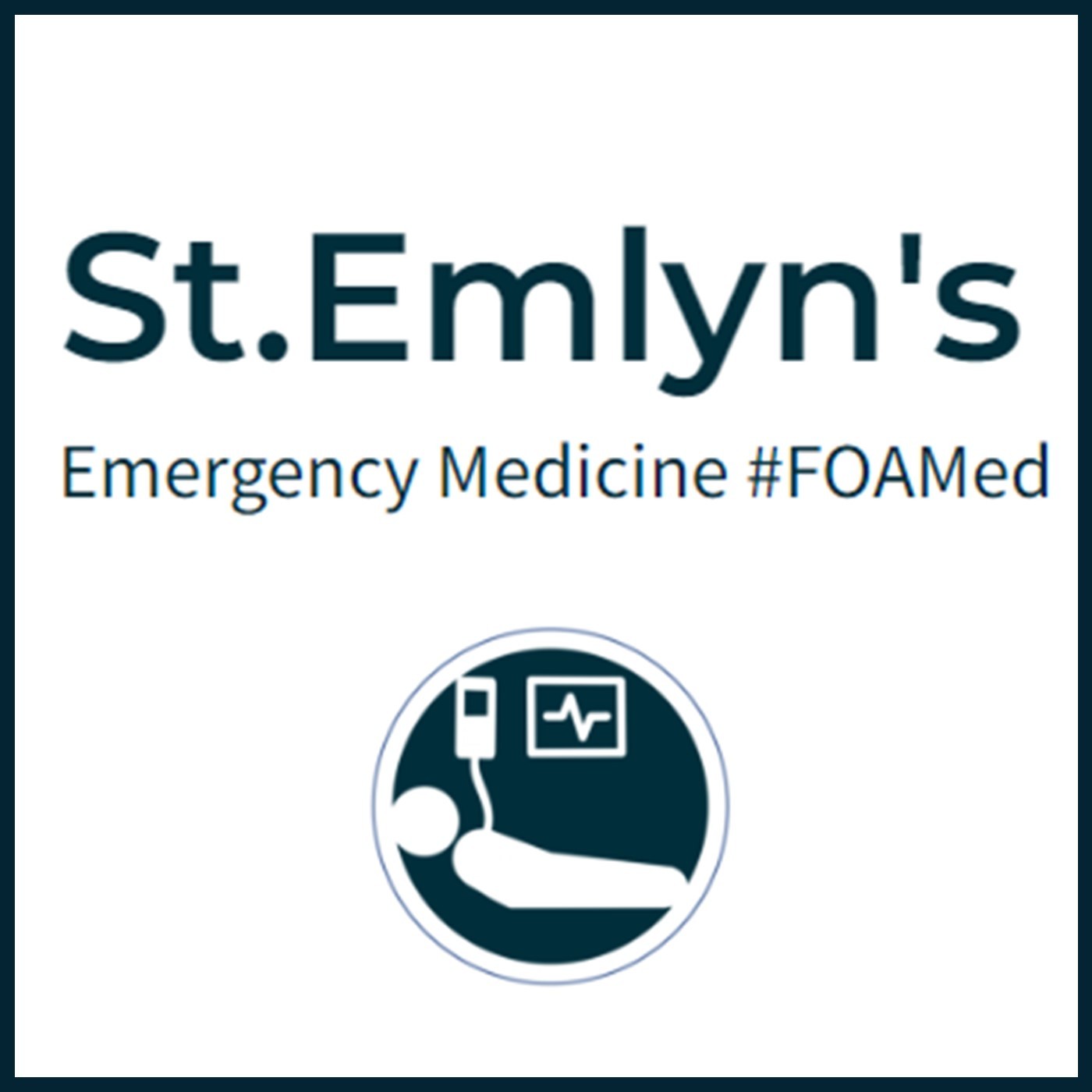
1.2M
Downloads
260
Episodes
A UK based Emergency Medicine podcast for anyone who works in emergency care. The St Emlyn ’s team are all passionate educators and clinicians who strive to bring you the best evidence based education. Our four pillars of learning are evidence-based medicine, clinical excellence, personal development and the philosophical overview of emergency care. We have a strong academic faculty and reputation for high quality education presented through multimedia platforms and articles. St Emlyn’s is a name given to a fictionalised emergency care system. This online clinical space is designed to allow clinical care to be discussed without compromising the safety or confidentiality of patients or clinicians.
Episodes
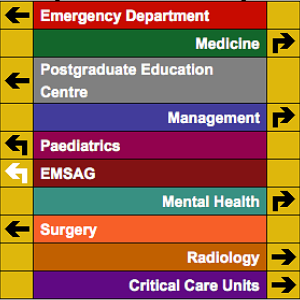
Saturday Feb 23, 2019
Ep 130 - Critical Appraisal Nuggets: p-values
Saturday Feb 23, 2019
Saturday Feb 23, 2019
Understanding P Values: A Comprehensive Guide for Clinicians
Welcome to St Emlyn's blog, where we delve into the complex world of P values—a crucial element in medical research. For emergency medicine clinicians, understanding P values is essential for interpreting study results and applying them effectively in clinical practice. This post aims to demystify P values and enhance your critical appraisal skills.
What Are P Values?
P values are a measure of the probability that an observed difference could have occurred just by chance if the null hypothesis were true. The null hypothesis generally states that there is no difference between two treatments or interventions. Thus, a P value helps us determine whether the observed data is consistent with this hypothesis.
The Null Hypothesis and Significance Testing
To grasp P values fully, we start with the null hypothesis. In any trial, we begin with the premise that there is no difference between the treatments being tested. Our goal is to test this null hypothesis and ideally disprove it, a process known as significance testing.
When we calculate a P value, we express the probability of obtaining a result as extreme as the one observed, assuming the null hypothesis is true. For instance, a P value of 0.05 suggests a 5% chance that the observed difference is due to random variation alone.
The Magic of 0.05
The threshold of 0.05 has become a benchmark in research. A P value below this threshold is often considered statistically significant, while one above is not. However, this binary approach oversimplifies statistical analysis. The figure 0.05 is arbitrary and does not imply that results just above or below this threshold are vastly different in terms of practical significance.
Clinical vs. Statistical Significance
Distinguishing between statistical significance and clinical significance is crucial. A statistically significant result with a very small P value may not always translate into clinical importance. For example, a large study might find that a new treatment reduces blood pressure by 0.5 millimetres of mercury with a P value of 0.001. While statistically significant, such a small reduction may not be clinically relevant.
Conversely, a clinically significant finding might not reach the strict threshold of statistical significance, particularly in smaller studies. Therefore, it's essential to consider both the magnitude of the effect and its practical implications in clinical practice.
The Fragility Index
The fragility index is an alternative measure that addresses some limitations of P values. It calculates the number of events that would need to change to alter the study's results from statistically significant to non-significant. This index provides insight into the robustness of the findings. Surprisingly, even large trials can have a low fragility index, indicating that their results hinge on a small number of events.
Moving Beyond 0.05
Recognizing the limitations of the 0.05 threshold, some researchers advocate for more stringent criteria, such as a P value of 0.02, particularly in large randomized controlled trials (RCTs). This approach aims to reduce the likelihood of false-positive results and improve the reliability of findings. However, it also raises the bar for demonstrating the efficacy of new treatments, which can be a double-edged sword.
Multiple Testing and Bonferroni Adjustment
A significant challenge in research is multiple testing. Conducting numerous statistical tests increases the probability of finding at least one significant result purely by chance. This issue is particularly relevant in exploratory studies where multiple outcomes are assessed.
One method to address this problem is the Bonferroni adjustment, which adjusts the significance threshold based on the number of tests performed. While this approach helps control the risk of false positives, it can be overly conservative and reduce the power to detect true effects. Therefore, it should be used judiciously.
Interim Analysis in Clinical Trials
Interim analysis is a crucial aspect of clinical trials, allowing researchers to assess the effectiveness or harm of an intervention before the study's completion. However, performing multiple interim analyses can increase the risk of false-positive findings. To mitigate this risk, researchers use techniques like P value spending functions, which adjust the significance threshold for each interim analysis.
Additionally, the number of interim analyses should be limited and pre-specified in the study protocol. This ensures that decisions to stop a trial early are based on robust evidence and not on arbitrary or opportunistic analyses.
Effect Size and Confidence Intervals
P values alone do not provide a complete picture of the study results. It's equally important to consider the effect size, which measures the magnitude of the difference between treatments. A small P value might indicate statistical significance, but without a substantial effect size, the clinical relevance of the finding remains questionable.
Confidence intervals (CIs) complement P values by providing a range within which the true effect size is likely to lie. A 95% CI means that if the study were repeated multiple times, 95% of the calculated intervals would contain the true effect size. CIs offer valuable context for interpreting P values and understanding the precision of the estimated effect.
Practical Tips for Interpreting P Values
- Understand the Null Hypothesis: Always start with a clear understanding of the null hypothesis and what the study aims to test.
- Look Beyond the P Value: Consider the effect size, confidence intervals, and clinical significance of the findings.
- Be Cautious with Multiple Testing: Recognize the increased risk of false positives with multiple comparisons and apply appropriate adjustments.
- Assess the Fragility Index: Use the fragility index to gauge the robustness of the study's findings.
- Consider Interim Analysis: Ensure that interim analyses are pre-planned and interpreted with caution to avoid bias.
- Question the Threshold: Remember that the 0.05 threshold is not a magic number. Interpret P values in the context of the study design, sample size, and practical implications.
Conclusion
P values are a fundamental aspect of medical research, but their interpretation requires a nuanced understanding. By considering the null hypothesis, clinical significance, effect size, and confidence intervals, we can make more informed decisions based on the data. As emergency medicine clinicians, our goal is to apply research findings judiciously to improve patient care.
We hope this deep dive into P values has clarified their role and limitations in research. Remember, the journey to mastering statistical concepts is ongoing, and continuous learning is key. If you have any questions or thoughts, please share them in the comments below. Happy appraising, and stay curious!
Comments (0)
To leave or reply to comments, please download free Podbean or
No Comments
To leave or reply to comments,
please download free Podbean App.